The Crucial Role of Vibration Monitoring in Enhancing Predictive Maintenance Outcomes and Better Asset Productivity
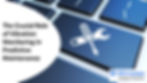
Within the article we will consider:
The role of vibration monitoring in the development and implementation of predictive maintenance strategies in various industries.
The technological advancements transforming the world of industrial machinery monitoring, with a focus on wireless vibration sensors, condition-based maintenance and real-time data analysis capabilities.
The intricacies of machine health management, highlighting the importance of fault detection, diagnosis and criticality rating to maintain optimal equipment performance.
Introduction to Predictive Maintenance and Vibration Monitoring
Predictive maintenance represents a proactive approach in asset management, leading the evolution of maintenance strategies with its ability to anticipate equipment anomalies and potential failures. Through the combination of artificial intelligence (AI), data analytics and Internet of Things (IoT) technology, predictive maintenance enables an optimal, data-driven decision-making process, ensuring operational efficiency and equipment reliability and mitigating unplanned downtime.
Vibration monitoring, which typically uses triaxial accelerometers (three axes) within wireless vibration sensors to collect data on equipment vibration, is one of the most important elements of predictive maintenance. Vibration monitoring considerably improves the effectiveness of predictive maintenance strategies. This monitoring process can detect abnormal condition, enabling early warning for machine faults and improving overall asset health management.
The vibration data collected is not just raw information but is transformed into actionable information through advanced analytics and machine learning algorithms. Such data analysis provides insightful diagnostics for equipment health, paving the way for a timely maintenance action, thus avoiding unexpected equipment downtime.
Elevate your asset management and optimise your operations: take our online IIoT opportunity evaluation today for actionable insights.
The importance of vibration monitoring in predictive maintenance resides in its ability to offer real-time condition monitoring, presenting a snapshot of the equipment health at any given moment. Additionally, by leveraging cloud computing and edge computing for industrial applications, continuous vibration monitoring systems can upload and analyse data remotely, ensuring real-time equipment monitoring and seamless remote condition monitoring.
Moreover, the combination of predictive maintenance and vibration monitoring allows asset performance management to go beyond traditional maintenance metrics. It introduces new elements like asset criticality, failure probability and total cost of ownership (TCO) into maintenance decision-making. In turn, this predictive maintenance approach reduces the cost of deferred maintenance, thereby minimising the direct maintenance cost.
Advancements in the industrial internet of things (IIoT) has given rise to an array of sensors and devices to form integral components of a condition monitoring system. These IoT enabled wireless sensors enhance the automated machine monitoring capabilities, further reinforcing industrial digital transformation.
With the onset of Industry 4.0 and Industry 5.0 technologies, the role of vibration monitoring in predictive maintenance has become more critical. Automated maintenance systems, equipped with the best wireless vibration sensors and machine learning for predictive maintenance, offer the dual benefits of improving maintenance productivity while reducing carbon footprint. These advancements in technology are essential in achieving Industry 5.0 sustainability goals.
What is Predictive Maintenance?
Predictive maintenance is an advanced maintenance strategy that uses data from various condition monitoring sensors and advanced analytics to predict equipment failure and defects before they occur. This approach differs from traditional reactive maintenance and preventative maintenance strategies which we outline below. Predictive maintenance is a subset of proactive maintenance, leveraging actionable information and machine learning algorithms to predict and avoid equipment failures.
Predictive maintenance relies heavily on IIoT (Industrial Internet of Things) and cloud computing to gather and analyse large volumes of data (big data) from multiple sources. The machine health data and equipment health data collected by IoT sensors like accelerometer sensors and temperature sensors provide the necessary insights for predictive maintenance actions.
Advanced technologies like artificial intelligence (AI), machine learning and digital twin technology are often integrated into predictive maintenance software with IIoT Data, making them a key part of a digital transformation strategy. AI and machine learning algorithms analyse the sensor data to identify patterns and trends, detect anomalies and forecast when equipment might fail (failure prediction). The digital twin, a virtual replica of a physical asset, allows for in-depth analysis and anomaly detection without affecting the actual asset.
Predictive maintenance offers several benefits such as reduced maintenance costs, minimised downtime, extended asset life, improved operational efficiency and a significant return on investment (ROI). Asset management solutions that include predictive maintenance strategies provide a comprehensive asset health management approach.
Understanding the Differences between Predictive Maintenance, Preventative Maintenance and Reactive Maintenance
As organisations strive to improve operational efficiency and reduce costs, the focus on maintenance strategies becomes paramount. There are three (3) main types of maintenance strategies: Predictive Maintenance, Preventative Maintenance and Reactive Maintenance.
Each of these strategies have their own benefits and drawbacks and the choice often depends on the nature of the equipment, available resources and the specific needs of the operation.
We discussed the definition of Predictive Maintenance above. Here are definitions of Preventative Maintenance and Reactive Maintenance.
What is Preventative Maintenance?
This is a routine maintenance strategy aimed at preventing the failure or degradation of equipment. It involves regular inspection, servicing and part replacements completed on a predefined schedule, regardless of the equipment's current health state, or if indeed the equipment requires maintenance at all. The aim of preventive maintenance is to keep equipment running smoothly and avoid costly, unexpected breakdowns.
What is Reactive Maintenance?
Also known as breakdown or run-to-failure maintenance, this approach involves waiting for equipment to fail or break down before taking action. Maintenance is carried out reactively and repairs or replacements are only completed when a machine has already malfunctioned or ceased to function. While this strategy may save on short-term maintenance costs, it will lead to higher costs in the medium-long run due to unexpected equipment failures, downtime and possible secondary damage.
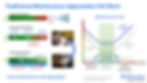
Each of these maintenance approaches has its unique benefits and challenges. Here is a comparative analysis.
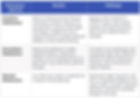
For more information on the impacts of deferred maintenance, please feel free to read of this article: "The True Cost of Deferred Maintenance".
A Fourth Evolving Maintenance Strategy. What is Prescriptive Maintenance?
Prescriptive Maintenance is a maintenance strategy that uses data analysis to anticipate potential equipment issues but also provide specific recommendations on actions to resolve these predicted problems, effectively optimising maintenance scheduling and operations.
Prescriptive Maintenance (PrM) goes beyond Predictive Maintenance (PdM) by not only anticipating equipment issues but also recommending corrective actions. The integration of AI and IoT, known as AIoT, greatly boosts prescriptive maintenance's capabilities. It collects real-time data from IoT sensors, analyses it with AI to identify potential faults and uses machine learning to suggest specific remedies. This could involve immediate maintenance or a future scheduled maintenance activity based on various factors like resources, operational needs and priority.
AIoT in prescriptive maintenance can further reduce downtime, extend equipment life and optimise resources, thereby enhancing operational efficiency and profitability over other noted maintenance strategies.
Condition Based Monitoring and How it Relates to Predictive Maintenance
Condition Based Monitoring (CBM) is an essential component of predictive maintenance. It involves the real-time monitoring of various parameters like vibration, temperature, acoustic emissions, humidity, etc., using condition monitoring sensors such as vibration sensors, temperature sensors, acoustic sensors, humidity sensors, etc.
CBM provides continuous insight into the asset's performance and health data, enabling data-driven decision-making. The data collected by condition monitoring sensors is analysed using advanced analytics and machine learning algorithms to identify any abnormal condition or performance degradation. This process, known as anomaly detection, allows for early warning of potential equipment failure.
The actionable information gathered from CBM feeds into the predictive maintenance system. This way, maintenance activities can be scheduled based on the actual condition of the equipment rather than on predetermined schedules. Such a strategy reduces unnecessary maintenance actions, saving time and resources and enhancing asset performance management.
Miniotec offers IoT technology that plays a crucial role in CBM, providing the necessary infrastructure for remote monitoring, wireless communication and data collection. The use of wireless vibration sensors and other wireless IIoT sensors simplifies the installation and reduces the cost associated with data acquisition in hard-to-reach areas or hazardous location installations.
By switching from time-based maintenance or reactive maintenance to a strategy that enables the equipment to talk and advise when is the best time for them to be maintained, predictive maintenance marks a paradigm change in asset management.
What is Vibration Monitoring?
Vibration monitoring, a critical element of best-practice asset management solutions and a key component in machinery health management, plays a pivotal role in modern maintenance strategies.
By constantly measuring and analysing the vibrational patterns of industrial equipment, this method enables early warning for potential anomalies or faults, ultimately preventing equipment failure and costly downtime. Through the use of advanced analytics, asset health data can be evaluated in real-time, providing actionable information for maintenance teams and driving data-driven decision-making processes.
The importance of vibration monitoring in predictive maintenance cannot be overstated. By identifying equipment anomalies at an early stage, organisations can reduce maintenance costs, improve operational reliability and maximise the lifetime of their assets. Vibration monitoring sensors, like the wireless vibration sensors offered by Miniotec, are used in a variety of industries, including Oil & Gas, Mining, Petrochemical and Renewable Energy and can be used in Pharmaceuticals and other Manufacturing processes. These devices are specifically designed to withstand hazardous area environments, high temperature, dust and humidity with the best versions having IP68 ratings.
The Three (3) Categories of Vibration Measurement
1.) Detection
Detection is the first step in the vibration measurement process. Detection utilises sensors for predictive maintenance such as vibration sensors and accelerometers to identify abnormal vibrations or noise levels in industrial machinery. These sensors provide high accuracy, high-frequency measurements that can signal a deteriorating machine condition or the need for asset maintenance. Thanks to recent advancements in technology, detection can now be performed remotely using wireless condition monitoring sensors. This remote condition monitoring capability has opened the door to continuous monitoring of assets in hard-to-reach areas or hazardous locations.
2.) Diagnosis
Diagnosis, the next step in vibration measurement, involves the use of data analysis and machine learning algorithms to determine the specific issue or fault causing the abnormal vibrations. By examining the diagnostic-quality waveform data collected by vibration monitoring sensors, specialists and/or automated systems can identify specific faults in bearings, pumps, gearboxes and other rotating equipment. Condition monitoring software, combined with cloud-based data analytics, can identify potential issues before they become major problems, aiding in the effective management of asset health and maintenance schedules.
3.) Prognosis
The final stage in vibration measurement, prognosis, leverages historical and real-time vibration monitoring data to predict future machine health. Artificial Intelligence and Machine Learning play a key role in this predictive maintenance strategy, using big data to model future equipment performance and potential failure points. The asset condition monitoring solution thus shifts from being reactive to proactive, enabling maintenance teams to plan for maintenance activities in advance, optimise resources and minimise unplanned production outages.
For some case study examples highlighting the success of predictive maintenance strategies that utilise wireless vibration sensor technology, please refer here.
Applications of Vibration Monitoring
Vibration monitoring has a wide range of applications across multiple sectors. It plays a critical role in asset management solutions, enhancing the effectiveness of asset maintenance and providing early indicators of equipment failure. Whether it's monitoring the bearing health in heavy machinery in mining operations, ensuring the operational efficiency of generators in the oil and gas industry, or overseeing the condition of pumps in petrochemical plants, vibration monitoring is instrumental.
By making use of remote vibration sensors and cloud-based remote monitoring, industries can not only detect but also diagnose and prognose issues well in advance, thereby reducing maintenance costs, preventing equipment downtime and achieving higher operational efficiency.
By leveraging the power of IIoT, machine learning and advanced analytics, vibration monitoring is transforming the industrial landscape, making it ready for Industry 5.0 and beyond.
Understanding Asset Categorisation
The Concept of Asset Categorisation / Asset Criticality
Asset Categorisation is a critical concept in asset management and predictive maintenance. It is a systematic approach to grouping and classifying an organisation's assets based on their criticality, usage and impact on overall operational efficiency.
This categorisation is an essential precursor to implementing any maintenance strategy, including predictive maintenance. By categorising assets, organisations can effectively prioritise maintenance actions, aligning resources and effort with the importance of each asset to the organisation.
Understanding asset categorisation is key for a data-driven decision-making process and leads to improved maintenance effectiveness, cost competitiveness and overall operational reliability. Categorisation can also guide decisions about deploying IIoT platforms for monitoring, selecting appropriate condition monitoring sensors and determining the level of investment in sophisticated solutions such as cloud-based data analytics and advanced machine learning algorithms.
The Three (3) Categories of Assets: 'A', 'B' and 'C'
Assets are typically classified into three (3) categories: 'A', 'B' and 'C'. The classification is generally based on parameters like asset criticality, cost of failure and the asset's contribution to operational performance and safety compliance.
Category 'A': Critical Assets
Category 'A' assets are those considered vital for the operation. These assets are often complex pieces of equipment, like heavy machinery in industrial applications or specific HVAC systems in commercial buildings. Failure of a Category 'A' asset could lead to significant operational disruption, safety risks, regulatory non-compliance and high maintenance costs.
These assets typically require sophisticated maintenance practices. IIoT vibration sensors, for example, may be used to continuously monitor the asset health, using Machine Learning for predictive maintenance though most Category 'A' assets utilise fix-wired systems.
The Cost of Failure for Category 'A' Assets
Given their criticality, the cost of failure for Category 'A' assets is usually high. This cost is not solely the direct maintenance cost, but also includes production downtime, unplanned production outages and potential penalties for regulatory non-compliance.
For example, in the Oil & Gas industry, a failed compressor could lead to substantial production losses, making it a Category 'A' asset. Therefore, a predictive maintenance strategy, supported by advanced analytics and artificial intelligence equipment monitoring, is typically used to avoid such failures.
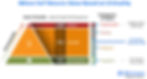
Category 'B': Essential Assets
Category 'B' assets are essential but not critical to the operations. These assets may not lead to a total shutdown if they fail, but their unavailability can still cause operational inefficiencies. Examples include auxiliary pumps or generators. Standby units are often available to replace these assets in the event of a failure.
The Role of Standby Units
Standby units play a significant role in managing Category 'B' assets. They act as a backup system and can be brought online immediately if the primary equipment fails. This reduces the impact of equipment downtime. These standby units, while not in regular use, should still be part of the maintenance schedule and their health monitored using appropriate equipment monitoring sensors because if they are equally faulty when initiated after the primary equipment fails, then your contingency of having a back-up system also fails.
Category 'C': Non-Essential Assets
Category 'C' assets, while not directly impacting operations, still contribute to the overall asset health management strategy. They might include assets like small pumps and motors, which do not halt production if they fail but can impact other areas or take the time of vital maintenance resources from more critical activities.
The Potential Benefits of Monitoring Category 'C' Assets
While Category 'C' assets might be deemed non-essential, monitoring them can still yield benefits. Consistent tracking of these assets can provide valuable data for maintenance optimisation. Their failures might not be operationally critical, but a high frequency of failures might indicate a larger issue that needs addressing. For such assets, condition monitoring technology like basic vibration monitoring or temperature sensors could be appropriate, providing sufficient asset health data without requiring the high investment of more sophisticated solutions.
For more discussion on Asset Criticality, read this article.
The Return on Investment (ROI) of Predictive Maintenance
Predictive maintenance plays an integral role in improving asset health, equipment reliability and overall operational efficiency. Its financial justification lies in the considerable cost savings it brings and its contribution to the digital transformation of the maintenance department.
The Direct and Indirect Costs of Equipment Failure
Equipment failure can lead to a plethora of direct and indirect costs. Direct costs, such as repair or replacement costs, are often easier to measure, but the indirect costs—like production downtime, lost revenue and a dip in customer satisfaction—are equally impactful.
Unplanned production outages due to equipment failure can lead to significant losses in all industrial sectors. The ripple effects include delayed deliveries, increased labour costs due to overtime work and a detrimental impact on a company's reputation. Moreover, equipment failure in critical infrastructure assets can lead to safety risks, regulatory compliance issues and even environmental resistance, amplifying the total cost of ownership.
How Predictive Maintenance Can Minimise Unforeseen Failures
Through condition monitoring systems like vibration analysis for IoT enabled predictive maintenance, asset condition monitoring solutions and sensors for predictive maintenance, asset owners will gather crucial asset health data and use it to provide actionable information. An effective predictive maintenance program uses these data-driven insights, advanced analytics and machine learning algorithms for anomaly detection, early warning and failure prediction, thereby minimising unforeseen failures.
Vibration monitoring predictive maintenance, for instance, employs wireless vibration sensors to monitor the status of assets continuously. This allows for real-time condition monitoring, detecting equipment anomalies and providing alerts before the occurrence of failure. Similarly, battery powered wireless IoT sensors for machinery, like a condition monitor vibration sensor or a temperature sensor, gather crucial data from hard to reach assets and hazardous location installations.
These tools, combined with big data analytics and AI-based predictive maintenance software, can transform equipment health monitoring by spotting potential issues well in advance, allowing for timely corrective maintenance. This in turn reduces the chances of catastrophic failures, improves equipment reliability and ultimately enhances asset performance management.
The Financial Justification for Predictive Maintenance
A well-implemented predictive maintenance program will lead to considerable cost reductions in the long run. Reduced manual effort, minimised equipment downtime, prolonged asset life, lower maintenance costs and optimised maintenance schedules are among the many advantages of condition monitoring that contribute to these savings.

Predictive maintenance strategies also help in Maintenance Planning, with a focus on prescriptive maintenance rather than costly reactive methods such as "operate to failure" maintenance. By preventing unexpected failures, predictive maintenance decreases the need for expensive emergency repairs and replacements, significantly reducing the direct maintenance cost.
Moreover, by reducing unscheduled downtime, predictive maintenance increases operational efficiency and production results, indirectly contributing to cost savings. IoT-enabled monitoring systems and wireless condition monitoring AI solutions also contribute to the energy efficiency of the assets, reducing carbon emissions and aiding in environmental compliance, further enhancing the ROI.
The Costs Associated with Setting up a Predictive Maintenance Program
Setting up a predictive maintenance program involves initial costs, all maintenance systems do, but the cost competitiveness and long-term benefits outweigh the upfront investment. These initial costs involve investment in hardware like wireless sensors for industrial applications, such as wireless acceleration sensors, wireless temperature and vibration sensors and software like asset management software and condition monitoring technology.
Also, investment in data acquisition systems, cloud-based remote monitoring and cybersecurity measures for a secure digital eco-system contribute to the setup cost. However, with the advent of versatile wireless technology, sophisticated solutions like wireless predictive maintenance sensors and cloud computing, the costs have significantly reduced, with many devices having long-life batteries and negligible installation costs.
For a more detailed analysis of ROI and IIoT, please read this detailed article titled: There Is More To ROI Calculations Than Simply Saving Manhour Costs
The Future of Vibration Monitoring in Supporting Predictive Maintenance Strategies
The advent of Industry 4.0 has seen vibration monitoring becoming an integral part of Equipment maintenance, moving from reactive approaches to more adoption of predictive maintenance technology and prescriptive analytics based strategies. This transition has not only led to improved machine health management but has also enhanced asset and equipment monitoring. The future growth of vibration monitoring in predictive maintenance strategies seems to hinge on several technological advancements.
Among these advancements is Energy Harvesting, a game-changer for machine vibration monitoring. The role of energy harvesting technology in powering machine vibration monitoring systems cannot be understated. It results in significantly improving the battery longevity of these systems. The recent development of triaxial wireless vibration sensors using energy harvesting technology is a great example.
Miniaturisation of Sensors is also a key trend. Smaller wireless condition monitoring technology will provide the same level of machinery protection through accurate data-driven maintenance, fault detection and fault diagnosis while maintaining a smaller footprint.
Edge Computing is an evolving technology that promises to enhance intelligent maintenance. This technology enables real-time condition monitoring by processing machine data on-site, thereby minimising latency. Industrial vibration monitoring systems are leveraging edge computing for more timely trending analysis and predictive maintenance as well as mitigating potential cyber security issues. Remote condition-based monitoring through remote vibration analysis is an application where edge computing is making a notable impact.
Finally, the advent of 5G and the impending arrival of 6G networks promise an exciting future for wireless condition-based maintenance and wireless machinery monitoring. These technologies are enabling real-time equipment monitoring and real-time vibration monitoring system deployment with 'super speed' data transfer. The high-speed data transmission capabilities of these networks will revolutionise fault detection, machine diagnostics, maintenance scheduling and maintenance technology as a whole.
The combination of these technologies will support an era of 'complete' maintenance, where wireless vibration monitoring equipment and systems will interact seamlessly with all machines, enabling continuous and automated machine optimisation. Wireless sensor solutions and wireless monitoring systems will be fundamental in delivering this vision, from vibration and temperature data collection to real-time diagnostics, with a focus on condition-based maintenance. This is the future of vibration monitoring in supporting predictive maintenance strategies.
In Summary
Predictive maintenance, empowered by vibration monitoring, provides a game-changing approach to asset management. Advanced analytics and machine learning algorithms drive this transformation, enabling the shift from traditional maintenance practices to condition-based maintenance (CBM) and predictive maintenance strategies.
Vibration monitoring is a critical component of any predictive maintenance strategy. The use of vibration sensors, such as triaxial accelerometer wireless vibration sensors, enables the real-time condition monitoring of machinery. This capability, when combined with advanced analytics, provides actionable information that enhances decision-making processes, thereby streamlining maintenance activities and reducing overall maintenance costs. The use of condition monitoring vibration sensors not only aids in early warning detection of abnormal conditions but also supports the reliability of machinery, contributing to increased asset health and longevity.
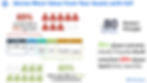
Machine learning and artificial intelligence further augment the capabilities of vibration monitoring, assisting in anomaly detection and offering predictive analytics that could identify potential failure points before they escalate to costly equipment failures.
The emergence of Industry 4.0 and the increasing adoption of the Industrial Internet of Things (IIoT) has a profound influence on the way we monitor and maintain industrial machinery. IIoT integration in business operations, alongside advancements in edge computing for industrial applications, enhances real-time data collection and analysis capabilities. This digital transformation, coupled with the use of cloud-based data analytics and the increasing reliance on IIoT for machine health monitoring, is a testament to the growing importance of technology in maintaining asset health.
Remote monitoring, facilitated by wireless technology, allows for continuous vibration monitoring of hard to reach assets or machinery operating in hazardous area environments, where manual inspection would be challenging or risky thus improves safety.
Investments in predictive maintenance and vibration monitoring technologies can result in considerable cost savings, driving a positive return on investment (ROI). By implementing predictive maintenance strategies, companies can avoid the high costs associated with equipment failure, reduce downtime and lower maintenance costs, all of which contribute to an improved bottom line.
Environmental considerations are also increasingly significant in asset management. Predictive maintenance strategies can contribute to energy efficiency and reducing carbon footprint, aligning industrial operations with sustainability goals.
In the world of IIoT and smart manufacturing, maintaining the security of operational data is paramount. Employing cybersecure protocols and ensuring cybersecurity is essential, particularly when dealing with sensitive vibration data, machine health data and other related information.
Finally, an effective predictive maintenance program is not only about implementing sophisticated solutions but also about ensuring easy configuration and installation, maximum performance, durability and environmental resistance of the chosen monitoring tools. Solutions like wireless condition monitoring system, IP68 specification devices and dust-proof and waterproof equipment, ensure that the monitoring system remains functional in all conditions, guaranteeing continuous measurement and providing superior data quality and quantity.
Overall, the advantages of predictive maintenance, including vibration monitoring, are manifold. From achieving cost competitiveness, optimising operational efficiency to enhancing asset performance, predictive maintenance marks a significant leap towards intelligent asset health management. The use of advanced analytics, artificial intelligence and machine learning, combined with IoT and wireless technology, make predictive maintenance a robust solution for the future of industrial maintenance.
By embracing the era of smart maintenance with AI and IIoT integration business strategies, we can realise the full potential of digital twin technology and advanced vibration analysis for IoT enabled predictive maintenance. So, let Miniotec support you as we venture into the exciting realm of predictive maintenance and vibration monitoring, underpinning the journey towards achieving operational excellence in the era of digital transformation.
Frequently Asked Questions
1. What is vibration monitoring? What is Vibration Analysis? Why is vibration monitoring important? What is the purpose of vibration analysis? Why is vibration analysis critical?
Vibration monitoring is the process that uses sensors to measure vibration levels in machinery and equipment. It involves monitoring the time waveform and frequency spectrum to identify potential issues. Vibration analysis is a method used to detect irregularities in machinery by interpreting the vibration frequency and levels of vibration. The primary purpose of vibration analysis is early detection of mechanical problems to prevent potential failure. It becomes critical because vibration analysis helps maintenance teams predict and address issues before they escalate, moving from reactive maintenance to a more proactive, predictive maintenance program. The use of vibration data can also guide vibration analysts and maintenance professionals in implementing the right maintenance work strategies and in supporting root cause analysis strategies.
2. What are the benefits of vibration monitoring?
The benefits of implementing vibration analysis and continuous monitoring include early detection of machinery defects, reducing downtime and improving system health. Monitoring also helps maintenance teams plan maintenance efficiently, offers numerous benefits in extending the lifespan of the machine’s parts and decreases the chances of unexpected failures. Additionally, based on vibration analytics, maintenance of industrial equipment can be optimised, resulting in significant cost savings.
3. What is the role of vibration monitoring in predictive maintenance? How can Vibration analysis help optimise predictive maintenance?
Vibration analysis is one of the pivotal parts of a predictive maintenance program. By measuring and analysing the different types of vibration levels, it provides insights into the condition of the machine.
Vibration analysis allows maintenance teams to identify vibration anomalies in numerous types of equipment, suggesting a potential issue. By analysing vibration data and focusing on vibration patterns when vibration occurs, predictive maintenance vibration strategies can be developed. This approach aids in shifting from preventive maintenance to predictive, ensuring timely replacement or service of parts and minimising unplanned downtime.
4. What is meant by predictive maintenance?
Predictive maintenance refers to the proactive approach of monitoring the condition and performance of equipment to predict when maintenance work should be performed. This approach ensures that maintenance is only performed when necessary, and it relies heavily on tools such as vibration analysis in maintenance, fixed vibration sensors and CMMS (Computerised Maintenance Management System) to anticipate potential failures.
5. What is the best way to measure vibration?
The best way to measure vibration is through the use of vibration sensors like those offered by Miniotec, predominantly accelerometers. These sensors measure the levels of vibration in equipment. The collected data is then analysed, often using Fast Fourier transform (FFT), to break down the vibration signals into their constituent frequencies. This vibration testing can identify potential mechanical issues based on vibration patterns.
6. What is Condition Monitoring vs Predictive Maintenance?
Condition monitoring is the process of monitoring specific conditions in machinery to ensure optimal operation. This might involve measuring and analysing factors such as temperature, lubrication and vibration levels. Predictive maintenance, on the other hand, uses the data obtained from condition monitoring (like that from vibration equipment) to predict when a machine will fail or require maintenance. Essentially, while condition monitoring offers a snapshot of system health, predictive maintenance uses that snapshot to predict future machinery health and potential failures.
7. What are common use cases for using a vibration sensor in predictive maintenance?
Vibration sensors are commonly used in monitoring rotating machines such as motors, pumps and gearboxes. They help maintenance teams detect imbalances, misalignments and wear in bearings. In the wide range of industries, from manufacturing to energy, the use of vibration sensors can pinpoint when maintenance or replacement is necessary, thus reducing downtime and enhancing asset reliability.
8. What early signs can Vibration Analysis help detect?
Vibration analysis can detect early signs such as imbalance in rotating machinery, misalignment, wear in bearings (inner race, outer race, bearing ball), gearbox issues and other potential mechanical faults. By focusing on vibration characteristics and analysing the frequency spectrum, potential problems can be identified and rectified before they lead to major breakdowns.
9. What kinds of issues does vibration analysis prevent?
Vibration analysis prevents a host of mechanical issues by offering insights into imbalances, misalignments, looseness and wear and tear in rotating machinery. By identifying these issues early, vibration analysis helps prevent major machinery failures, costly repairs, extended downtimes and potential safety hazards.
10. What is asset reliability and how does Vibration monitoring relate?
Asset reliability refers to the capability of equipment and systems to perform their intended functions without failure over a specified period. Vibration monitoring is an essential tool in ensuring asset reliability. By consistently monitoring and analysing the vibrations of equipment, potential issues can be identified and addressed early on, ensuring the equipment remains reliable and operational for its intended lifespan. This makes it a useful tool for improving overall productivity and efficiency in various industries.
Let us know your thoughts?
Stay safe.
Best;
About Miniotec:
Miniotec is a digital consulting and technology solutions provider, dedicated to supporting companies in their digital transformation journeys. Established by a group of experienced engineers, we emphasise the harmonious integration of people, processes and technology. Our team has a rich history of working across various sectors, from energy and resources to infrastructure and industry. We are trusted by the world's largest miners, oil and gas giants, utility companies and even budding start-ups and believe in the transformative power of the Industrial Internet of Things (IIoT) and its role in unlocking valuable data insights. Through IIoT, we aim to facilitate better decision-making, enhance operational activities and promote safer work environments. At Miniotec, our goal is to guide and support, ensuring every digital step is a step forward.
VibrationMonitoring
PredictiveMaintenance
IndustrialIoT
SmartManufacturing
MachineHealthManagement
WirelessMonitoringTechnology
DigitalTransformation
Miniotec