Optimising Equipment Reliability - Managing the Waddington Effect with Industrial IoT (IIoT) and Artificial Intelligence (AI)
- Miniotec - Intelligent Solutions
- Mar 16, 2024
- 15 min read
Updated: Apr 30, 2024
Exploring the Shift from Manual to Predictive Maintenance: The Role of IIoT and AI in Modernising Industrial Reliability
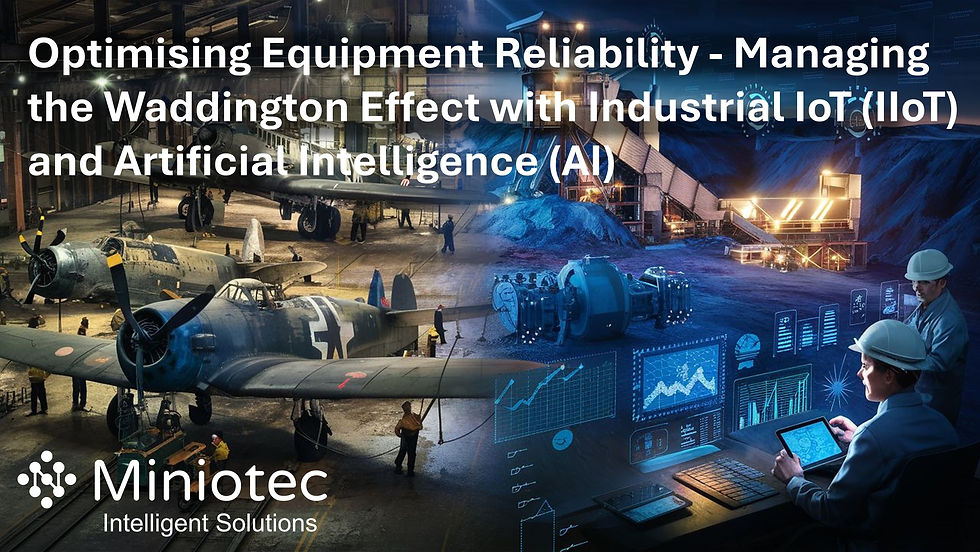
Introduction
Organisations across energy, mining, manufacturing and other heavy industries are on a quest for peak performance and dependable equipment reliability. To achieve this, adopting cutting-edge technology has become an essential component of industrial success. However, amidst this technological evolution, industries encounter a peculiar and contradictory challenge—the Waddington effect. This concept, a phenomenon that challenges the very tenets of traditional maintenance strategies, raises a critical question: Could the integration of the Industrial Internet of Things (IIoT) and Artificial Intelligence (AI) not just tackle, but also turn the tables on this effect, propelling predictive maintenance and asset life cycle management to new heights? By venturing into the synergy between these advanced technologies, this article explores their potential to transform the way we approach current maintenance practices, offering a glimpse into a future where maintenance strategies are not just reactive, but proactively intelligent.
Key Takeaways - The Transformative Power of Industrial IoT (IIoT) and AI in Managing the Waddington Effect.
Understanding the Waddington effect and its implications on traditional equipment maintenance protocols.
Exploring how IIoT leverages connectivity and data for advanced predictive maintenance strategies.
Insights into AI innovation and its transformative role in early anomaly detection and maintenance optimisation.
The evolution of asset life cycle management through the integration of AI and IoT technologies.
Discovering the benefits of weaving IIoT and AI into the strategies of industry practices to boost equipment reliability.
A concise review of how technology helps to transcend conventional limits, ensuring greater operational efficacy and resilience.
Understanding the Waddington Effect in Equipment Maintenance - The Origins of Predictive Maintenance (PdM)
In the segment of equipment maintenance, the Waddington effect represents a complex challenge to achieving sustained performance and extending the life of essential machinery. This effect captures the intriguing contradiction between expected equipment reliability and the increased likelihood of failure following maintenance activities. Although not widely recognised, its significance touches upon various aspects of operational efficiency, including maintenance strategies and the integration of technological advancements. For industry professionals looking to navigate these challenges, understanding the Waddington effect is crucial. By leveraging machine learning and AI, they can counteract its potential negative impacts and enhance the durability and reliability of equipment.
What is the Waddington Effect? - The Origins and Implications
The Waddington effect, a seemingly paradoxical phenomenon, has its roots in the complex world of equipment maintenance and reliability. Consider the following scenario: you are working hard to keep a piece of machinery in top condition, but your efforts appear to be doing more harm than good. This is the essence of the Waddington effect. It was first observed and developed by Conwy Lloyd Morgan in the early 20th century, then later named after C.H. Waddington, a British biologist who delved deeper into this intriguing occurrence. (You can read more about the Waddington Effect and its origins here).
This effect illustrates the unexpected correlations between routine maintenance tasks—those we perform to keep machines running smoothly—with increased breakdowns and equipment malfunctions. It is somewhat like watering a plant with the good intention of nurturing its growth, but overwatering it instead, which leads to root rot. In the world of maintenance, this counterintuitive outcome means that the very actions intended to prolong equipment life and enhance operational effectiveness might actually be the catalyst for failure.
The implications of the Waddington effect are far-reaching, especially when considering the financial impact. Maintenance operations, designed to avert failure, end up leading to increased costs and both scheduled and unscheduled downtime. This phenomenon raises significant concerns about the long-term reliability and cost-effectiveness of mission-critical equipment across industries. Understanding the Waddington effect is essential for professionals looking to strike the right balance between necessary maintenance and the potential for inadvertently accelerating equipment wear and tear.
How Maintenance Programs and Inspections Impact Equipment Reliability
Inspection and maintenance efforts, which were previously thought to be resilient in their ability to avoid machine malfunctions, are now being examined through the lens of the Waddington effect. Analyses indicate that maintenance interventions might unintentionally introduce new faults or exacerbate existing weaknesses, bringing into question the very practices designed to bolster reliability. Synthesising historical evidence with present studies highlights the need for evolving strategic approaches to anticipate and navigate these unintended consequences.
Maintenance Approach | Traditional Expectation | Waddington Effect Impact |
Regular Inspections | Preventative measure to reduce failures | Potential increase in frequent, minor malfunctions |
Replacement of Parts | Extending equipment lifespan | Unwittingly inducing component incompatibilities |
System Calibration | Maintaining optimal operational parameters | Risk of miscalibration and subsequent performance issues |
Lubrication and Cleaning | Ensuring smooth operation and longevity | Introduction of contaminants or over-lubrication incidents |
The Waddington Effect on Various Maintenance Aspects
The delicate balance between maintaining equipment and avoiding breakdowns has never been more critical. Addressing the Waddington effect requires a sophisticated and meticulously developed approach to inspection and maintenance—a strategy that is crucial for industries aiming to maintain operational readiness and prevent equipment failures.
Adapting to Industry 4.0: IIoT - Transforming Maintenance
The advent of Industry 4.0 has ushered in a period of unparalleled transformation in the industrial sector, with the adoption of IoT solutions at the forefront. Traditional maintenance is being transformed into intelligent, predictive operations, aided by advances in Industrial IoT (IIoT) technologies. The seamless integration of automation and increased connectivity has begun to redefine the paradigms of maintenance methodologies, infusing them with efficiency and a proactive stance that was previously undreamed of in legacy systems.
IIoT platforms serve as the cornerstone for this evolution, embodying the convergence of machine-to-machine communication, data analytics and cloud-based solutions. These technological force multipliers offer the capacity to collect and analyse data across a vast array of remote equipment, sometimes in hazardous locations, in real-time. It is not just about gathering data however; it is about distilling it into actionable data driven insights that drive predictive maintenance technologies, allowing industries to pre-emptively address potential disruptions before they mushroom into costly downtimes.
The facilitation of automation in equipment monitoring and diagnostics, ensuring a continuous array of data that feeds into predictive algorithms.
Enhanced connectivity that provides a comprehensive view of operational health, from a single machine to an entire fleet, irrespective of geographic constraints.
The application of predictive maintenance technologies that analyse data patterns to forecast wear and tear, leading to timely interventions.
Deployment of cloud-based solutions for centralised data processing, optimising resources and enabling scalability of maintenance operations.
With the application of IIoT, industrial enterprises have seen a notable shift in maintenance performance. The proactive measures enabled by these technologies are not mere incremental improvements away from mundane activities but are rapidly becoming vital pieces of a competitive strategy within the Industry 4.0 framework. As we delve further into this discussion, we will elucidate on the specific impacts and benefits of IIoT's role in predictive maintenance and how it is transforming the way industries operate and maintain their equipment.
AI-Based Predictive Analytics - Moving from Preventive Maintenance to a Path to Maintenance Optimisation
In an era where operational excellence hinges on efficiency and foresight, AI-based predictive analytics stands out as a critical technological enabler. Combining these advanced analytics with regular maintenance activities creates an ideal environment for unprecedented optimisation and reliability in industrial settings.
Leveraging Machine Learning Algorithms for Anomaly Detection
Central to this transformation is the implementation of machine learning, an AI technology that thrives on data to improve decision-making over time. Through its capability to ingest and analyse vast datasets, machine learning algorithms become adept at recognising patterns and pinpointing outliers. This anomaly detection is critical, as it signals potential equipment failures before they escalate into costly downtimes or hazardous situations. Consider the following comparative analysis:
Maintenance Aspect | Without Machine Learning | With Machine Learning |
Anomaly Identification | Manual inspection, prone to human error | Automated with high precision, constant |
Data Quantity Analysis | Limited to humanly-manageable datasets | Analysing vast, complex datasets efficiently |
Response Time | Slower due to manual data processing | Rapid analysis, near-real-time feedback |
Operational Downtime | More frequent and prolonged | Reduced significantly through pre-emptive actions |
The Benefits of AI and Machine Learning on Various Maintenance Aspects
New to Artificial Intelligence (AI)? Read our article on "Why Every Company Needs a Corporate AI Policy". You can also try our free GPT that helps you develop your own tailored AI Policy framework. A good first step to developing an AI Policy for your organisation.
Transitioning from Reactive to Proactive Maintenance with AI
The transition from a reactive to a proactive maintenance model is already underway, steered by the forward-thinking capabilities of AI. By incorporating AI-based predictive analytics, organisations can evolve from a stance of reacting to failures as they occur, to anticipating and preventing them. This shift not only curtails interruptions but also paves the way for a more streamlined and cost-effective maintenance workflow - a contrast that yields palpable benefits, including:
Significant reduction in unplanned downtime, enhancing overall productivity.
Extension of machinery life through timely, condition-based interventions, leveraging machine learning and AI for predictive maintenance. This approach now enables your 'dumb' assets the ability to talk.
Resource allocation to critical tasks, improving the efficacy and morale of maintenance crews.
The synergy of AI-based predictive analytics, machine learning and proactive maintenance strategies formulates an integral foundation for maintenance optimisation. Such intelligent systems are quickly becoming indispensable in driving operational efficiency and securing a competitive edge in the fast-paced industrial landscape.
The Role of Connectivity: 5G and Cloud-Based Solutions in Maintenance
The rapid evolution of 5G technology has presented new heights of connectivity critical to industrial maintenance. This advanced network infrastructure is instrumental in enabling real-time monitoring and the swift transmission of the voluminous data essential for data-driven maintenance. With higher bandwidth and lower latency, 5G stands at the forefront of a connectivity revolution, allowing maintenance systems to transition to cloud-based platforms that scale on demand and support complex computational tasks with ease.
Cloud-based solutions are indispensable as they host powerful analytics tools and facilitate centralised data management, making way for innovative maintenance approaches that are both adaptive and predictive in nature. This pivotal shift towards cloud computing and storage, in tandem with the arrival of 5G, translates into cohesive, actionable insights derived from wireless condition monitoring systems distributed across vast industrial landscapes.
Unprecedented speeds and reliability in data transfer offered by 5G enhances the capability of predictive systems, providing real-time feedback for maintenance decisions.
Cloud platforms serve as scalable repositories for equipment data, improving accessibility and collaboration across different departments and locations.
Combined, these technologies support an ecosystem that converges on maximum uptime and efficiency, thanks to more intelligent and responsive maintenance protocols.
The synergy of 5G and cloud-based solutions equips industry professionals with the tools to address the immediate needs of equipment while strategically planning for future maintenance—all without being tethered to a wired network. The efficiency of operations and predictive maintenance is vastly improved, underscoring the critical role of seamless connectivity in keeping industrial processes at peak performance.
Connectivity is the lifeblood of modern maintenance strategies. The combination of 5G and cloud-based platforms is creating an era where digital resilience translates into operational resilience, unveiling new vistas for maintenance efficiency.
Moving forward, the implementation of 5G networks and robust cloud-based solutions will remain pivotal to the development of maintenance practices that are seamless, efficient and ultimately transformative in their ability to leverage data for operational excellence.
Implementing Condition-Based and Predictive Maintenance Practices
In the sphere of industrial maintenance, a significant shift is underway, transitioning from traditional scheduled maintenance to a dynamic, condition-based approach. This shift is driven by an operational research-driven urge to enhance efficiency and prevent unexpected machine downtime. By harnessing the power of sensor data and embracing condition-based maintenance, and even energy centred maintenance (ECM), industries can predict and pre-empt maintenance issues more effectively.
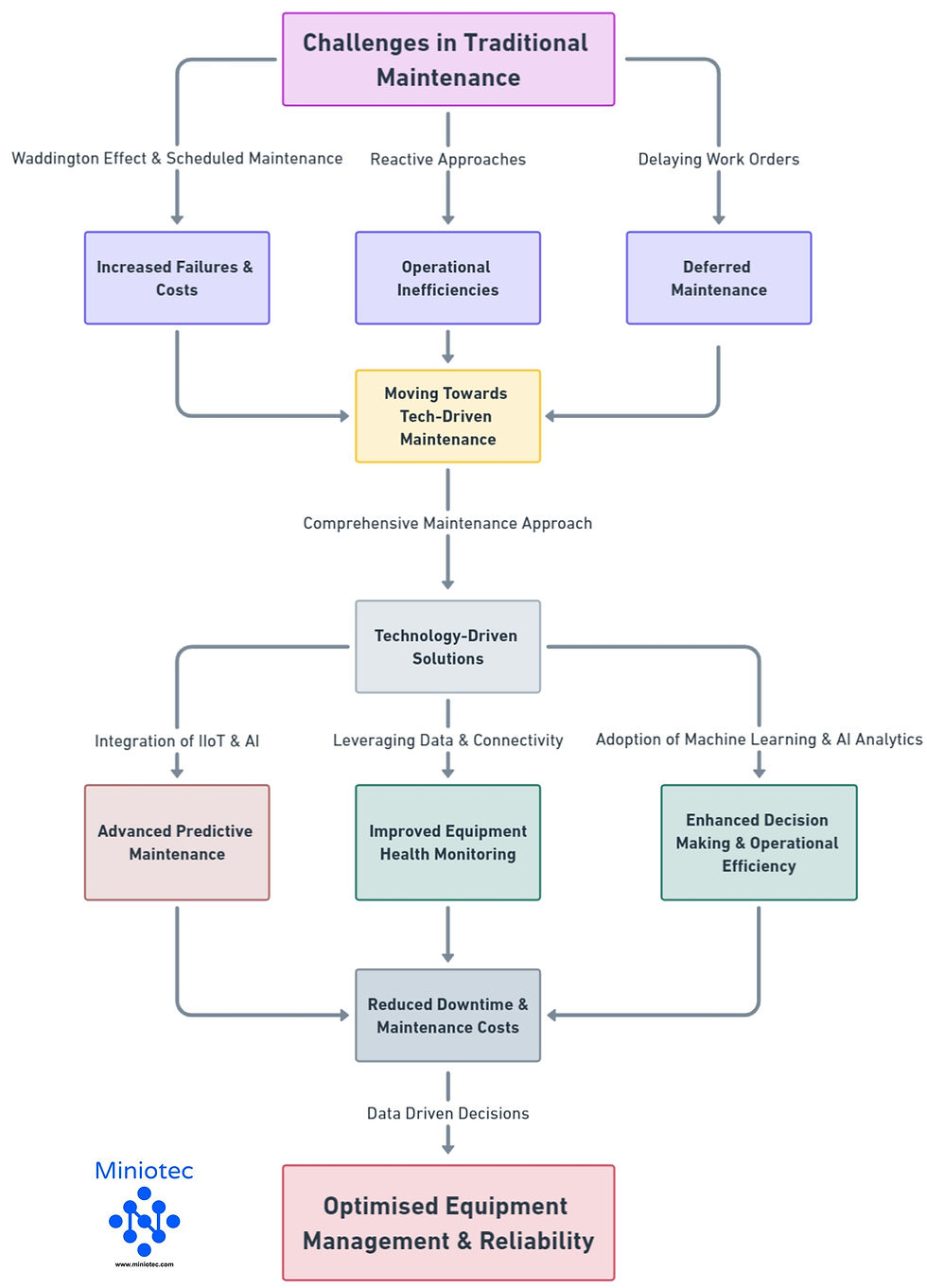
From Scheduled to Condition Monitoring: A Shift in Methodology
The era of sticking to a fixed maintenance schedule is fading. Today, maintenance strategies are increasingly tailored to the real-time condition of equipment, adapting maintenance efforts to the live health status of machinery, a strategy referred to as condition-based maintenance. Instead of relying on time intervals or usage metrics, machinery is now monitored in real-time, with maintenance activities initiated based on actual equipment health and performance indicators. This dynamic method leverages the latest in predictive maintenance solutions, optimising inspection cycles and maintenance events to reduce downtime and increase equipment reliability.
Leveraging Sensor Intelligence for Smarter Maintenance through More Informed Maintenance Decisions
Central to the transformative approach of condition-based and predictive maintenance is the strategic utilisation of sensor data. Sensor technologies, such as those used for vibration monitoring in bearing and gear systems or tracking the condition of lubricants, provide critical, real-time insight into equipment health. These real-time data points are invaluable for making informed maintenance decisions, facilitating a shift from reactive to proactive and predictive care that can significantly extend asset life and performance. Predictive maintenance solutions aggregate and analyse sensor data, from oil and gas motor condition monitoring to real-time fluid condition analysis and electrical system diagnostics, to detect potential issues long before they escalate into serious problems.
This nuanced approach marks a departure from the over maintenance tendencies highlighted by the Waddington effect, advocating for a more informed and efficient strategy. Rather than arbitrary servicing that could inadvertently lead to increased wear and tear, the focus now is on maintenance that is guided by the actual condition of the equipment. This shift not only helps in sidestepping the unintended consequences of over maintenance but also aligns with the ultimate goal of ensuring that machinery is maintained just in time, based on its self-reported health state.
An example of the evolution in maintenance decision-making using sensor data can be visualised in the following comparative table:
Scheduled Maintenance Approach | Condition-Based Maintenance (CBM) Approach |
Based on predetermined intervals (Preventative Maintenance) | Triggered by real-time data and alerts |
Frequent unnecessary inspections (Preventative Maintenance) | Inspections aligned with actual need |
Potential for premature maintenance (Preventative Maintenance) | Maintenance timed with actual wear and tear |
Reactive to failures (Reactive Maintenance) | Proactive prediction of potential issues |
The Evolution in Maintenance Decision-Making Using IIoT Sensor Data
The incorporation of real-time, sensor-generated data has reoriented the maintenance landscape towards a more efficient, predictive model. Today's maintenance processes are becoming more intelligent, leveraging the exact timing of condition-based monitoring and the forecasting capabilities of predictive maintenance solutions to maintain optimal equipment performance and extend operational lifespan.
Data-Driven Strategies: Achieving Actionable Insights Through Equipment Data Analysis
The advent of sophisticated predictive maintenance technology has paved the way for data-driven strategies that enhance equipment reliability and performance. But the ability to achieve actionable insights is highly contingent upon the quality and analysis of the data at hand. Utilising precise and accurate data enables industries to make more informed decisions, ensuring the reliability and longevity of their machinery.
The Importance of Data Accuracy and Quality in Predictive Maintenance
To unlock the full potential of predictive maintenance, the accuracy and quality of collected data cannot be overemphasised. Inaccuracies or gaps in data can lead to misinformed strategies that compromise equipment health and productivity. Maintaining high standards of data accuracy is, therefore, a cornerstone in developing reliable predictive maintenance models that provide actionable insights and preventative solutions.
Organising Massive Amounts of IoT Data for Effective Analysis
As modern equipment continues to integrate an array of sensors and devices, industries are faced with the challenge of organising massive amounts of data. Effective data analysis processes are critical for transforming large amounts of information into valuable insights. A structured approach to IoT data management not only speeds up the analysis process but also improves the precision of predictive maintenance initiatives.
Data Parameter | Challenges in Handling | Methods for Effective Analysis |
Volume | Storage and processing of vast data sets | Database partitioning, data warehousing |
Velocity | Real-time analytics for fast data streams | Stream processing, time-series analysis |
Variety | Diverse data types and sources | Data integration tools, Extract, Transform, Load (ETL) processes |
Veracity | Ensuring data quality and accuracy | Data cleaning, validation procedures |
Value | Extracting actionable insights from data | Advanced analytics, machine learning algorithms |
Data Management and Maintaining Data Integrity is Significantly Important For Successful Predictive Maintenance Outcomes
With the implementation of advanced data analysis procedures, industries can effectively navigate through the complexities of IoT data to extract actionable insights that drive predictive maintenance technology forward. By prioritising data accuracy and constantly refining analysis methods, businesses cement their capabilities to deliver optimised maintenance strategies and secure a competitive advantage within their respective sectors.
The Waddington Effect in Navigating Maintenance in Energy, Mining and Heavy Infrastructure
In the demanding industries of energy, mining, manufacturing and heavy infrastructure, equipment reliability is critical. The Waddington effect poses a unique challenge, emphasising the delicate balance between routine maintenance and the unintended consequences that can jeopardise equipment effectiveness. This phenomenon not only escalates maintenance expenses but also pushes the need for postponing essential upkeep, impacting the operational readiness critical to these industries.
Addressing the Waddington effect requires a deep dive into its broader implications, particularly how it affects operational efficiency. Beyond the immediate rise in maintenance costs, the real concern lies in the postponement of necessary maintenance — deferred maintenance — which can lead to a domino effect of reliability and availability issues. For sectors where downtime equals significant financial and operational losses, ensuring equipment readiness is not just beneficial; it's essential for an organisation's bottom line, and sometimes, survival.
Strategically managing the trade-offs between maintaining immediate operational capability and fostering long-term equipment reliability demands a thorough evaluation of maintenance practices affected by the Waddington effect. This approach involves examining several key aspects:
Consideration | Impact on Operational Efficiency | Long-term Reliability Outcome |
Maintenance Frequency | Increased downtime may initially reduce efficiency | Potential for higher reliability with better maintenance approaches |
Deferred Maintenance | Short-term operational needs met, risking future reliability | Greater chance of equipment failure and escalated maintenance costs |
Maintenance Cost Impact | Immediate cost savings from postponed or deferred maintenance | Overall increase in long-term expenses and potential for major overhauls |
Readiness Impact of Faulty Equipment | Reduced equipment effectiveness, risking project timelines | Decreased asset life and compromised functionality |
The Trade-offs Between Maintaining Immediate Operational Capability and Fostering Long-term Equipment Reliability Demands a Thorough Evaluation of Maintenance Practices.
In essence, effectively tackling the Waddington effect entails a nuanced understanding and strategic planning in maintenance operations. Adopting a comprehensive maintenance strategy that balances immediate operational demands with the goal of prolonged equipment lifespan is crucial. Such a balanced approach is key to achieving sustained operational efficiency and reliability, navigating the intricate dynamics of maintenance in the energy, mining and heavy infrastructure sectors. Through thoughtful analysis and proactive maintenance planning, utilising AI and IIoT strategies, organisations can overcome the challenges posed by the Waddington effect, ensuring their operations remain robust and resilient.
Wireless Vibration Monitoring Systems: Optimising Industrial Equipment Health and Reliability
Industrial environments are rapidly advancing with the integration of innovative technologies in equipment health monitoring. Wireless vibration monitoring systems have emerged as a key player in this technological leap, redefining the approach towards predictive maintenance in industrial equipment.
Advances in Bearing Vibration Sensor Technology
With a focus on remote condition monitoring, the advances in bearing vibration sensor technology are reshaping the efficiency and accuracy of predictive maintenance. Modern wireless sensors are becoming increasingly sensitive, capable of detecting the slightest anomalies in equipment performance. The enhanced diagnostic and trending capabilities provided by these sensors offer an early-warning system for potential equipment failures, thus paving the way for avoiding costly downtime and reducing maintenance overheads.
These sophisticated sensors not only detect common faults but can also trend data over time to predict the possible lifespan of machinery bearings. This critical advancement in sensor technology allows for the meticulous planning of maintenance schedules and resource allocation, ensuring that machinery operates at optimum performance levels.
Remote Monitoring: Keeping a Pulse on Equipment Anytime, Anywhere
Remote monitoring stands as a testament to the flexibility and resilience of wireless vibration analysis systems. Adopting a holistic approach to machinery health, these systems deliver continuous surveillance and data collection capabilities, to equipment managers without requiring a physical presence at the site of the machinery. Regardless of location, technicians can now monitor equipment health remotely, fostering an environment where preventive measures can be enacted swiftly upon the detection of an anomaly.
The scalability of remote monitoring systems provides maintenance teams with a comprehensive overview of equipment conditions across multiple sites, all from a centralised location. Implementing these systems empowers industries with the advantage of intervening before the occurrence of critical breakdowns, fortifying the ethos of wireless predictive maintenance and vibration predictive maintenance.
Real-time alerting mechanisms ensure swift identification of potential issues, minimising equipment downtime.
Data-driven insights derived from remote monitoring analytics enhance decision-making for maintenance planning.
Scalability and flexibility of wireless systems facilitate the expansion of monitoring networks without extensive infrastructure changes.
Wireless vibration monitoring systems are at the forefront of a preventative maintenance revolution, offering unprecedented visibility into the health and performance of industrial equipment. As these technologies continue to evolve, they will undoubtedly play a pivotal role in the future of industrial machinery maintenance and reliability.
In Summary
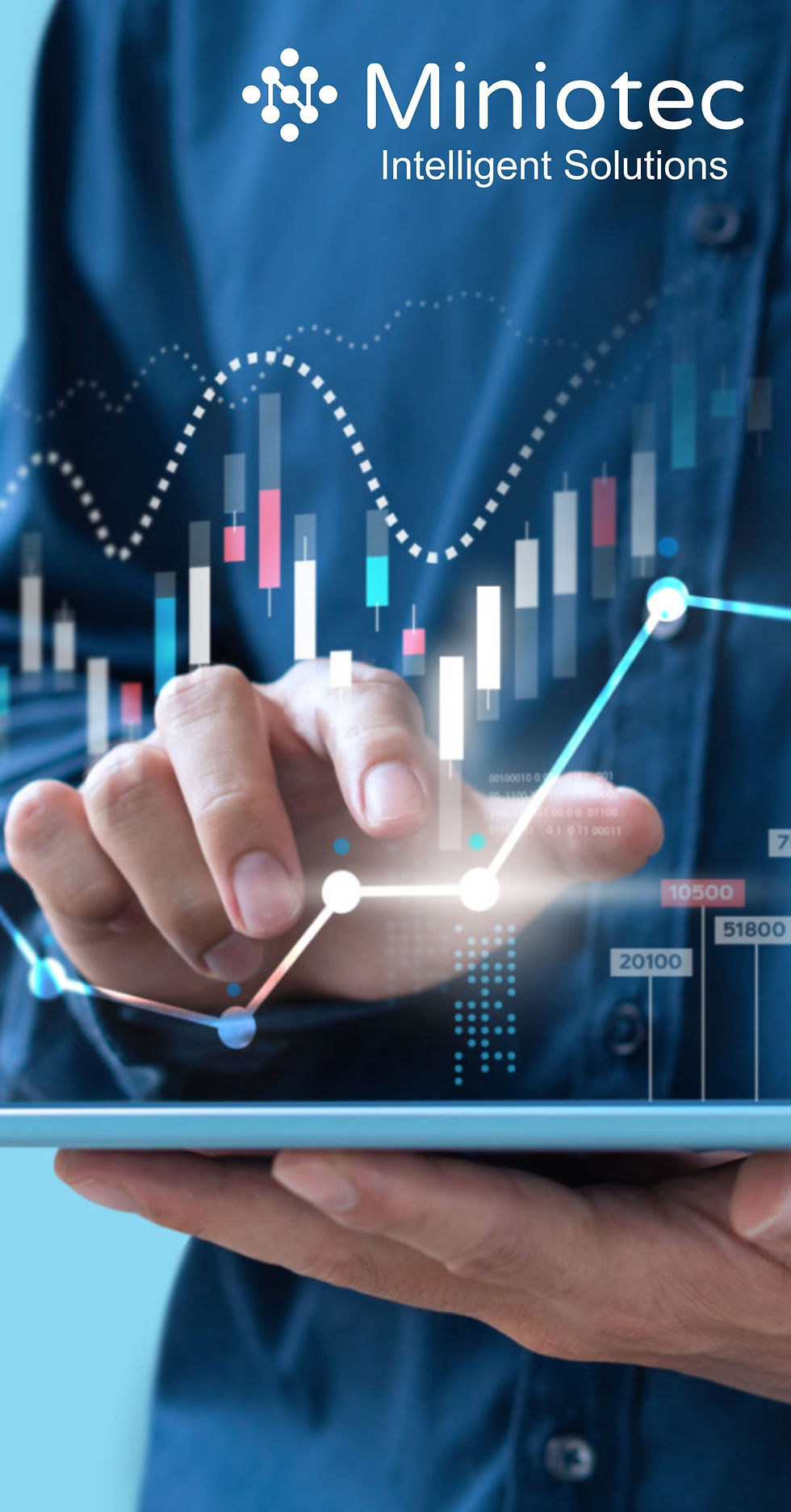
As we define the course of industrial innovation, it is clear that integrating IIoT and AI has become the critical component for improved equipment management.
In this article we have explored the multifaceted role these technologies help in counteracting the elusive Waddington effect and move the predictive maintenance paradigm towards a more astute and responsive domain. From real-time analytics to advanced machine learning algorithms, IIoT and AI represent a dynamic solution that drives the improvement of equipment reliability and life cycle management.
Integrating IIoT and AI for Enhanced Equipment Life Cycle Management
The seamless convergence of IIoT and AI technologies is not just a trend but a strategic transformation. This amalgamation paves the way for an interconnected system that can anticipate maintenance needs, prescribe prompt corrective actions and enhance operational efficiency. By bridging the data-driven acumen of AI with the extensive connectivity of IIoT, industries are now equipped to surmount the Waddington effect, ensuring a resilient and reliable equipment management framework that prolongs asset lifespan and mitigates unnecessary downtime.
Future Trends in Maintenance: What's Next After Managing the Waddington Effect?
Looking towards the future horizon, the trajectory of maintenance technology is set to ascend even further beyond the realms of the Waddington effect management. Anticipated future trends suggest a rapid advancement of AI sophistication, including the potential for autonomous maintenance systems and self-healing machinery, Industry 5.0, Digital Twins and Generative AI supported maintenance. As the predictive maintenance evolution continues to unfold, we stand on the cusp of realising truly intelligent operations. It is this ongoing innovation in integrating IIoT and AI that promises a renaissance through AIoT, where the emergence of technology is inextricably linked to the overriding objective of industrial resiliency and peak operational efficiency.
To stay ahead in the rapidly evolving landscape of industrial maintenance, we invite you to embrace the cutting-edge capabilities of IIoT and AI. Begin your journey towards achieving unparalleled equipment reliability and efficiency by integrating these transformative technologies into your maintenance strategy today.
Frequently Asked Questions
Q1. How does the Internet of Things (IoT) enhance predictive maintenance in heavy industries?
IoT technology enables organisations to adopt predictive maintenance solutions by providing real-time data from sensor-based devices. This data, when analysed, allows for condition-based maintenance, reducing downtime and maintenance costs significantly.
Q2. What role does IIoT play in transforming maintenance strategies within an organisation?
The Industrial Internet of Things (IIoT) integrates seamlessly with existing control systems, leveraging automation and data analytics to shift from reactive to predictive and even prescriptive maintenance methodologies. This transition not only optimises maintenance activities but also enhances the uptime and reliability of equipment.
Q3. Can you explain how artificial intelligence and machine learning contribute to monitoring and predictive maintenance?
AI and machine learning analyse massive amounts of data generated by IIoT devices to identify patterns and predict potential failures before they occur. This AI-based approach allows for more accurate condition monitoring and predictive analytics, leading to smarter maintenance practices.
Q4. What advantages do cloud-based platforms offer for predictive maintenance in Industry 4.0?
Cloud-based platforms provide a scalable architecture that supports the storage and analysis of vast datasets from connectivity-enabled devices. This facilitates end-to-end predictive maintenance solutions, leveraging real-time data and predictive analytics for effective decision-making and automation of maintenance activities.
Q5. How does 5G technology impact the efficiency of IoT and IIoT devices in predictive maintenance?
5G technology enhances the quality of data collection and transmission, enabling the handling of time series data and the management of massive amounts of data with minimal latency. This improved connectivity ensures that organisations can monitor operating parameters more efficiently, making real-time condition monitoring and predictive maintenance more effective and reliable.
Special thanks to Tony Nitchov for his expert insights that greatly enhanced our discussion on IIoT, AI and predictive maintenance.
We welcome your insights and experiences.
Stay safe.
Best;
About Miniotec:
Miniotec is a digital consulting and technology solutions provider, dedicated to supporting companies in their digital transformation journeys. Established by a group of experienced engineers, we emphasise the harmonious integration of people, processes and technology. Our team has a rich history of working across various sectors, from energy and resources to infrastructure and industry. We are trusted by the world's largest miners, oil and gas giants, utility companies and even budding start-ups and believe in the transformative power of the Industrial Internet of Things (IIoT) and its role in unlocking valuable data insights. Through IIoT, we aim to facilitate better decision-making, enhance operational activities and promote safer work environments. At Miniotec, our goal is to guide and support, ensuring every digital step is a step forward.
Digital Transformation
Predictive Maintenance
Industrial IoT Integration
AI in Maintenance
Waddington Effect
Equipment Reliability Optimisation
Smart Maintenance
Miniotec